All posts by Farid Qamar
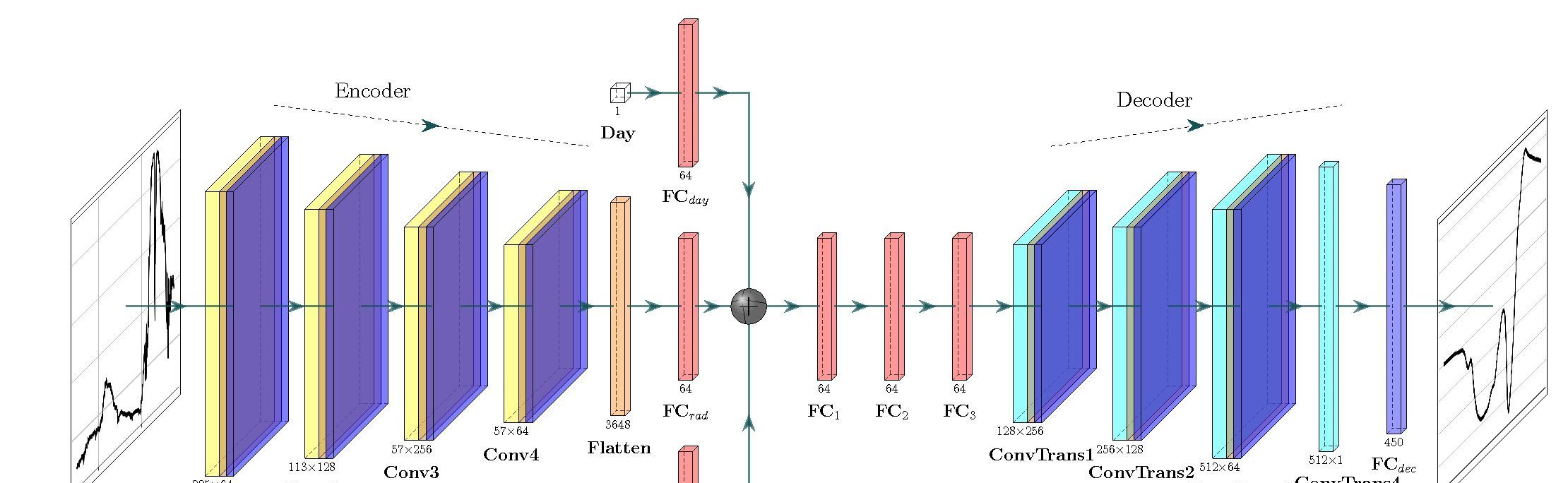
Atmospheric correction of vegetation reflectance with simulation-trained deep learning for ground-based hyperspectral remote sensing
We propose a framework for obtaining the vegetation spectral reflectance from at-sensor spectral radiance, which relies on a time-dependent Encoder-Decoder Convolutional Neural Network trained and tested using simulated spectra generated from radiative transfer modeling.
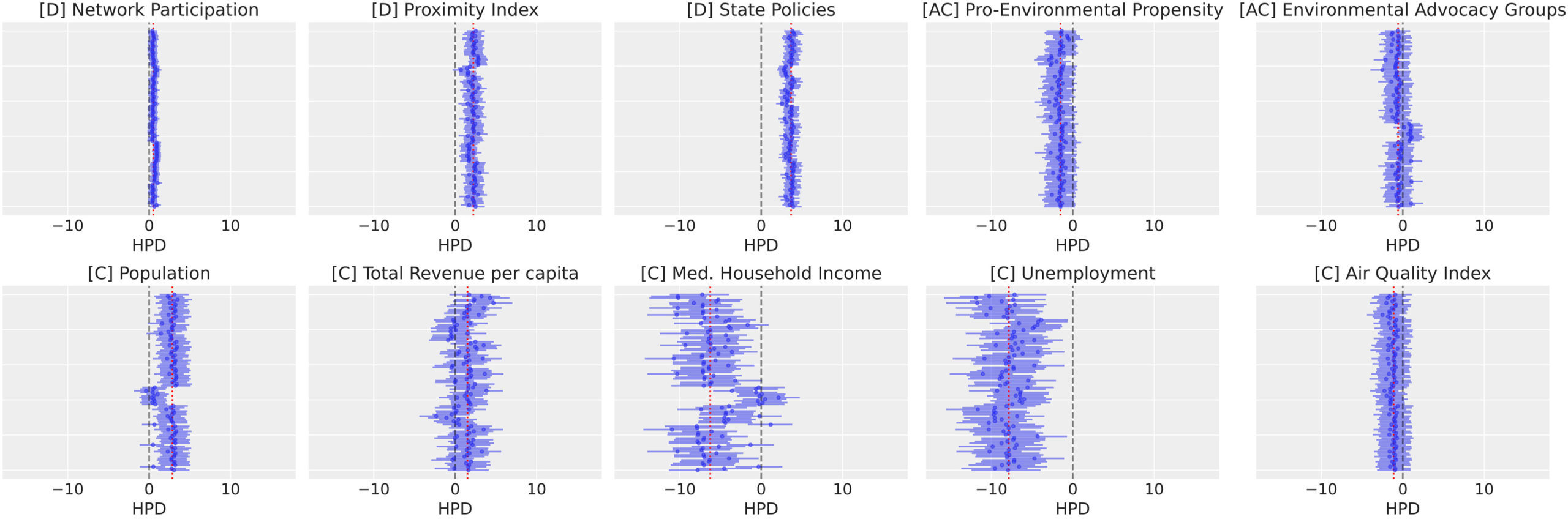
Covariance in policy diffusion: Evidence from the adoption of hyperlocal air quality monitoring programs by US cities
In this work, we explore the influence of multiple diffusion mechanisms: learning, imitation, and coercion; policy mobility factors; and internal determinants on the likelihood of hyperlocal air quality monitoring program (HAMP) adoption by large cities in the US with a population >300,000 over the past decade.
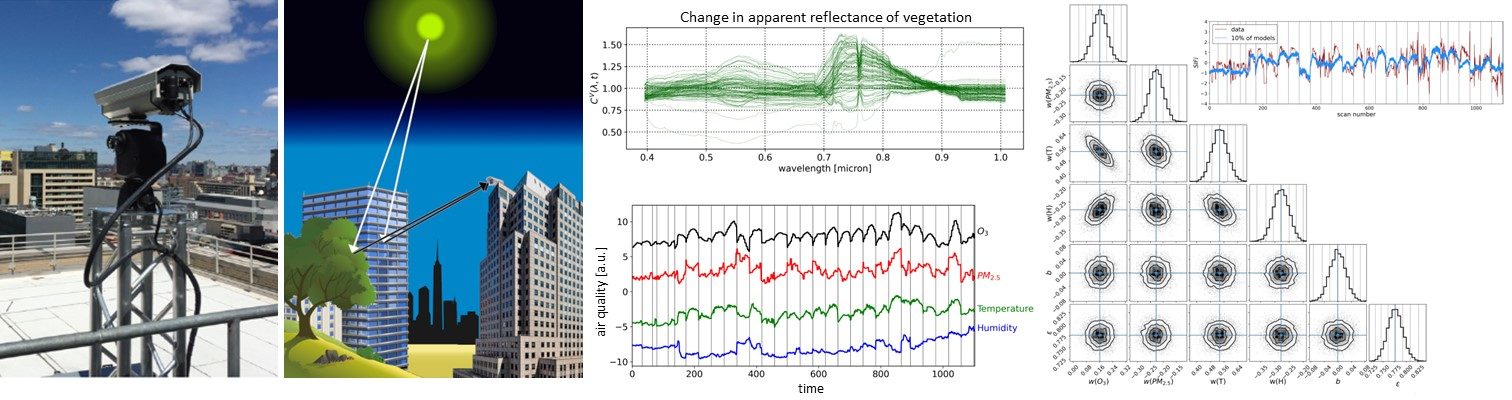
The Impacts of Air Quality on Vegetation Health in Dense Urban Environments: A Ground-Based Hyperspectral Imaging Approach
We examine the impact of changes in ozone (O3), particulate matter (PM2.5), temperature, and humidity on the health of vegetation in dense urban environments, using a very high-resolution, ground-based Visible and Near-Infrared (VNIR, 0.4–1.0 μm with a spectral resolution of 0.75 nm) hyperspectral camera deployed by the Urban Observatory (UO) in New York City.
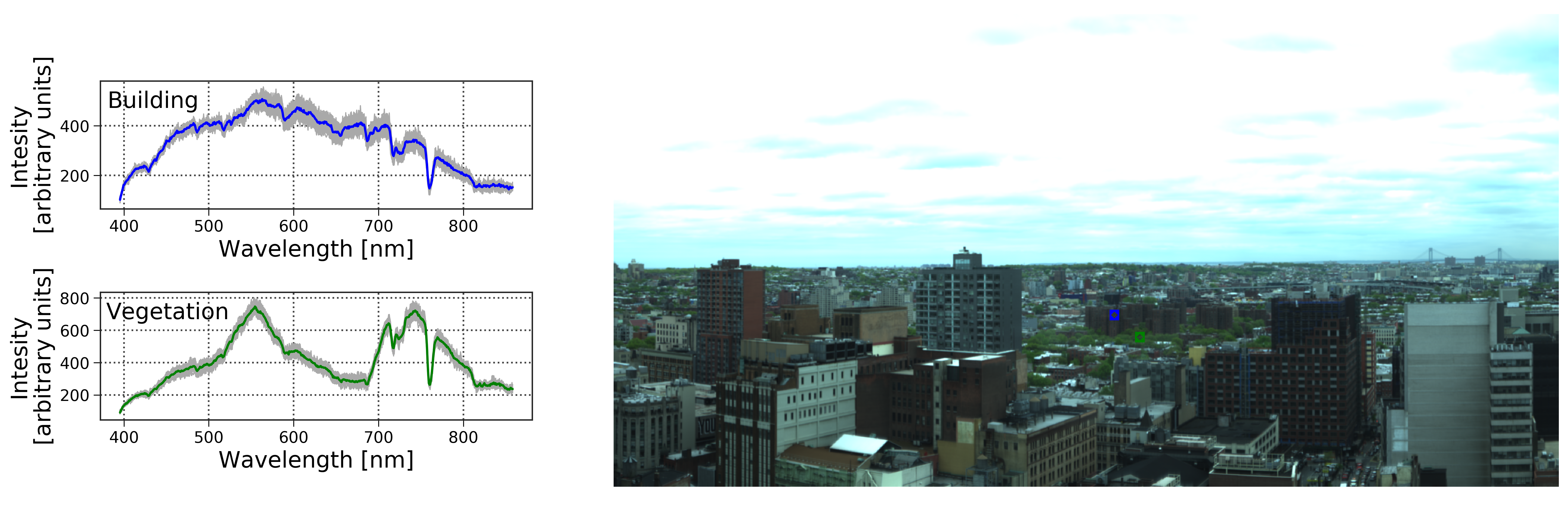
The Effects of Atmospheric Modeling Covariance on Ground-Based Hyperspectral Measurements of Surface Reflectance
This paper presents a novel framework for estimating the covariance and uncertainties of atmospheric parameters and the reflectance spectra of urban surfaces in high-resolution ground-based hyperspectral images.
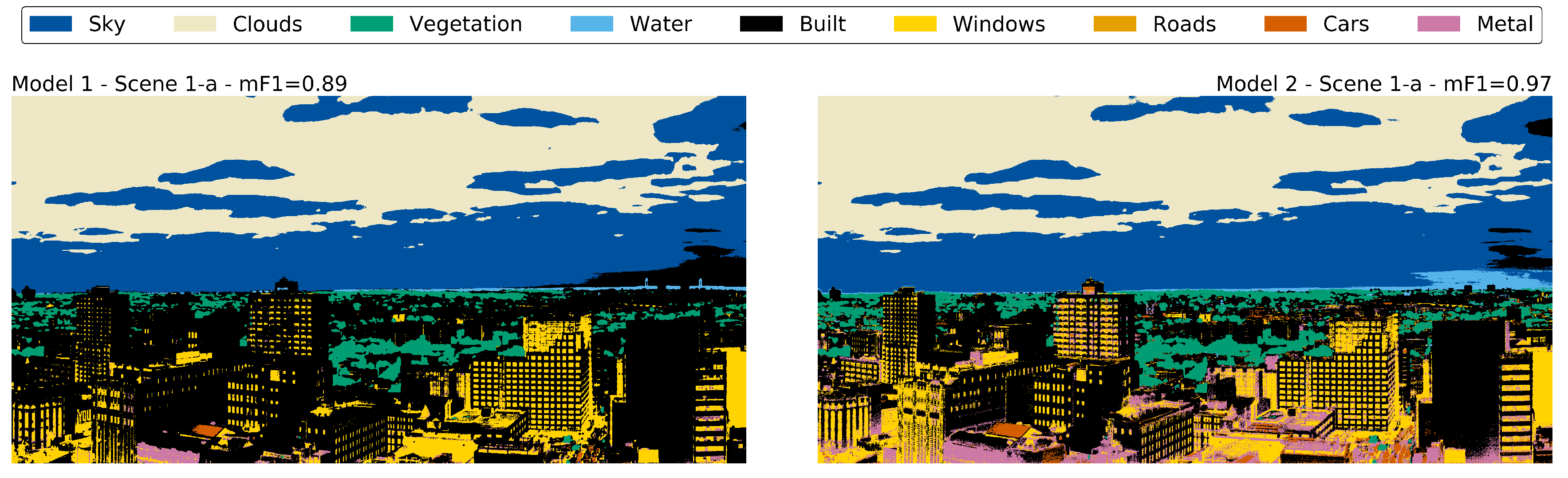
Pixel-Wise Classification of High-Resolution Ground-Based Urban Hyperspectral Images with Convolutional Neural Networks
Using ground-based, remote hyperspectral images from 0.4–1.0 micron in ∼850 spectral channels—acquired with the Urban Observatory facility in New York City—we evaluate the use of one-dimensional Convolutional Neural Networks (CNNs) for pixel-level classification and segmentation of built and natural materials in urban environments.
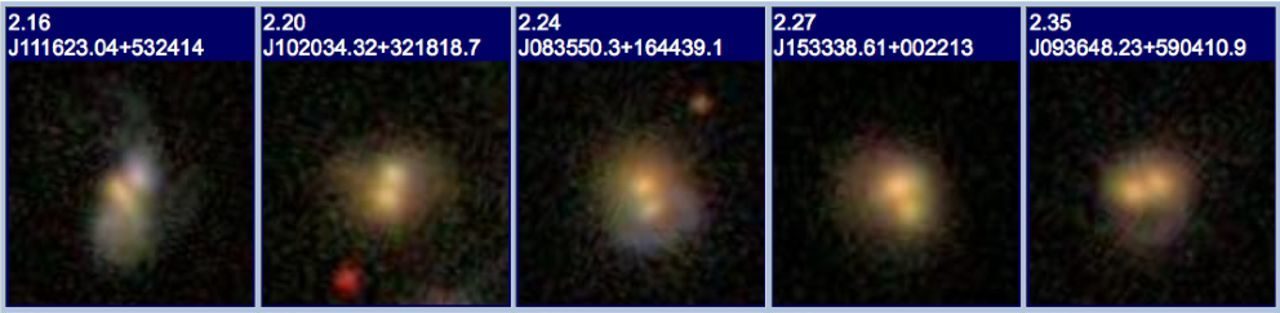
Galaxy pairs in the Sloan Digital Sky Survey – XI. A new method for measuring the influence of the closest companion out to wide separations
We describe a statistical approach for measuring the influence that a galaxy’s closest companion has on the galaxy’s properties out to arbitrarily wide separations.
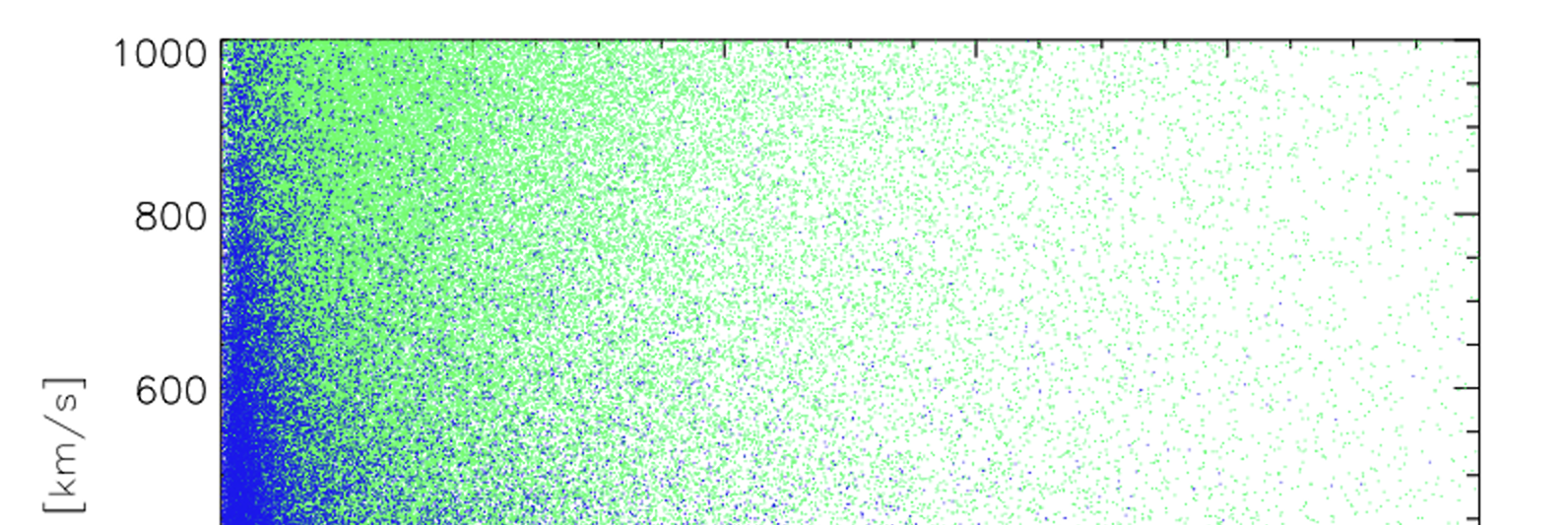
Environmental Effects on Interacting Galaxy Pairs in the SDSS
The cause of varying galaxy interaction outcomes in different environments is investigated using a sample of over 505,000 Sloan Digital Sky Survey Data Release 7 galaxies at 0.01 < z < 0.20. The aim is to distinguish the large-scale environmental effects on galaxies from the small-scale interaction effects in order to identify whether interaction mechanisms vary in different environments, or whether different galaxy populations produce the various outcomes in different environments.
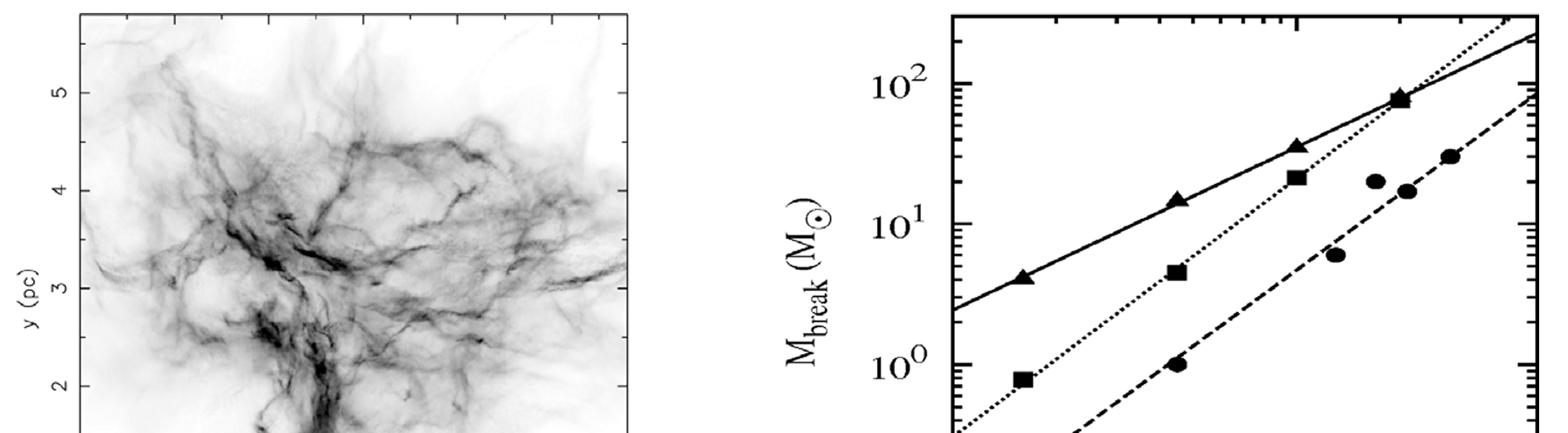
Analogues of Cores and Stars in Simulated Molecular Clouds
We use images derived from collapsing, turbulent molecular cloud simulations without sinks to explore the effects of finite image angular resolution and noise on the derived clump mass function.